RelEYEance: Gaze-based Assessment of Users’ AI-reliance at Run-time
Zekun Wu, Yao Wang, Markus Langer, Anna Maria Feit
Proc. ACM on Human-Computer Interaction (PACM HCI), 9(ETRA16), pp. 1–18, 2025.
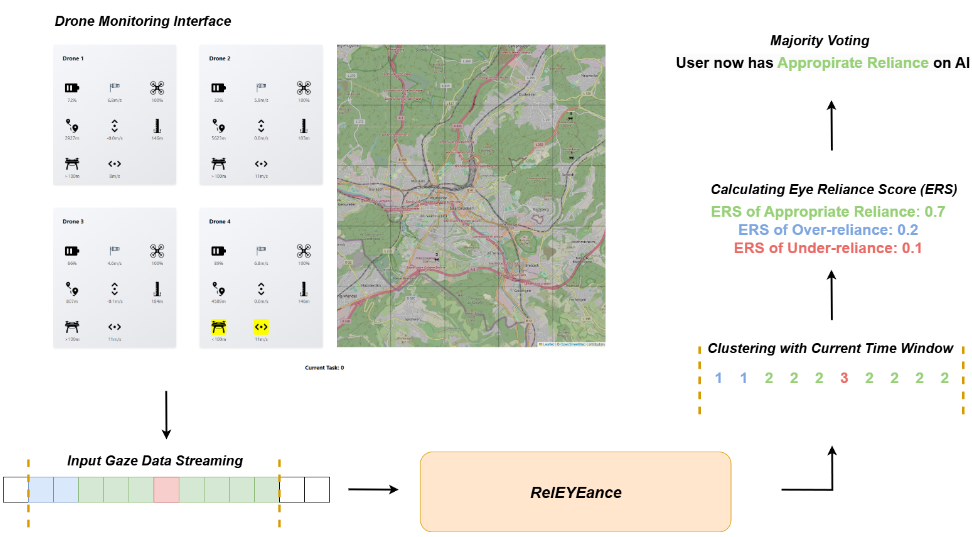
Abstract
In time-critical detection tasks,such as drone monitoring, a key condition for users to effectively leverage AI assistance is to find an appropriate trade-off between making fast decisions and verifying AI suggestions, which we refer to as appropriate user reliance. However, assessing such reliance is often oversimplified by focusing solely on task outcomes, potentially overlooking whether users properly verify AI messages. We collected eye-tracking data from an AI-assisted monitoring task and developed a gaze-based reliance model: RelEYEance, to assess the extent of user reliance on AI-suggested alarms. We found that gaze patterns related to verification behaviors distinguish between appropriate reliance, over-reliance, and under-reliance, influencing task performance. We validated our model in a second user study, showing it can reliably detect users’ over- and under-reliance at run-time, which could be used e.g. for issuing intervention messages. The results demonstrate the potential for real-time human-AI reliance assessment, facilitating adaptive reliance calibration.Links
doi: 10.1145/3725841
Paper: wu25_etra.pdf
BibTeX
@article{wu25_etra,
title = {RelEYEance: Gaze-based Assessment of Users’ AI-reliance at Run-time},
author = {Wu, Zekun and Wang, Yao and Langer, Markus and Feit, Anna Maria},
year = {2025},
journal = {Proc. ACM on Human-Computer Interaction (PACM HCI)},
doi = {10.1145/3725841},
volume = {9},
number = {ETRA16},
pages = {1--18}
}