AI-assisted Semi-automatic Labelling of Eye Contact Data
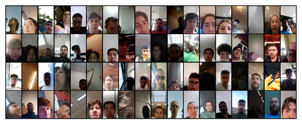
Description: With recent advancements in sensing and, in particular, camera technology, it has become possible to collect large-scale datasets of human behaviour. However, manually annotating and labelling such data is not only tedious but also time consuming. Many existing tools and methods have been designed to annotate single or individual samples, yet it is often the case that images share similarities and often the same annotation label.
In this project, we will work on a novel method for semi-automatic labelling of eye contact data. We will use the existing EMVA dataset (Bâce et al. 2020) and propose methods to group images that, e.g., share the same head pose or gaze direction and, hence, share the same eye contact label. This project will involve exploring state-of-the-art methods for head pose estimation (Ruiz et al. 2018) and appearance-based gaze estimation (Zhang et al. 2015). Image source: www.emva-dataset.org
Supervisor: Mihai Bâce
Distribution: 30% Literature, 10% Data Preparation, 30% Implementation, 30% Analysis and Evaluation
Requirements: Familiarity and interest in machine learning
Literature: Bâce, Mihai, Sander Staal and Andreas Bulling. 2020. Quantification of users' visual attention during everyday mobile device interactions. Proceedings of the 2020 CHI Conference on Human Factors in Computing Systems (CHI).
Ruiz, Nataniel, Eunji Chong and James M. Rehg. 2018. Fine-grained head pose estimation without keypoints. Proceedings of the 2018 IEEE/CVF Conference on Computer Vision and Pattern Recognition Workshops (CVPRW).
Zhang, Xucong, Yusuke Sugano, Mario Fritz and Andreas Bulling. 2015. Appearance-based gaze estimation in the wild. Proceedings of the 2015 IEEE Conference on Computer Vision and Pattern Recognition (CVPR) .